Rising costs, shrinking margins, and supply chain issues are problems that impact us daily and manufacturers are looking for any and all ways to do more with the processes and systems that they already have. One option that is often overlooked for getting more out a process is improvements in multivariable process control.
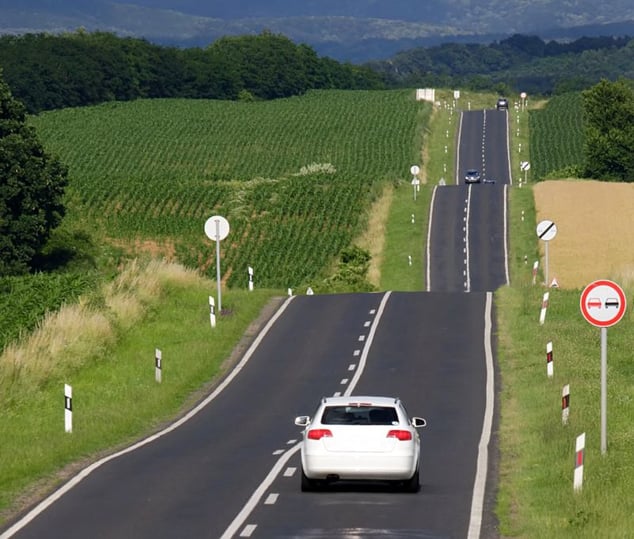
A multivariable process is, simply put, any that has more than one input and/or more than one output required for proper control and for which multiple setpoints are involved. The complexity arises when the setpoints in your system are cross-coupled with one another, meaning that a change in one setpoint affects the process variable of another (and potentially vice versa). Other complexities occur with processes that have long time delays or high order dynamic responses. Examples of multivariable systems include:
- Distillation column
- Reactor control (e.g. trying to control both level and concentration)
- Petroleum refining
- Natural gas recovery
- In-line blending
- Power systems
If the interactions of the cross-coupled variables are too strong, basic process control (e.g. PID Loops) may not provide you with the most efficient and predictable system that can optimize your yields, maximize your production output, and decrease your energy use.
Chances are good that you already have practical experience with multivariable control – your morning commute. At its simplest, driving is pressing on the accelerator to go and the brake to stop. When you depress the accelerator, the engine helps bring your car up to the desired speed setpoint (the speed limit, of course). As you approach the setpoint, you ease off the accelerator to the point where the engine has just enough gas to keep you at the speed limit. Simple, right?
Of course, not all roads are flat. To maintain your speed on a hill, you must generally depress the accelerator before the car has reached it. This feed forward approach means that the car’s speed does not take a dip in the beginning of the incline. In multivariable process control, many variables are measured, and decisions are made based on some, or all, of this data. In our driving example, there are many other factors that can affect the position of the accelerator – traffic ahead, an intersection with a traffic light, a stopped school bus, or changing weather conditions to name a few. With so many variables possible, we can see why it has taken so long to realize advances in self-driving vehicles.
A Brief Overview of How Multivariable Process Control Works
The most common automated multivariable control technology is model-predictive control (MPC), a control technique that uses dynamic process models to predict the future response of the process. The models used in MPC are developed during plant tests to identify the dynamic and steady state effects on the control variables (CVs) when manipulated variables (MVs) or disturbance variables (DVs) are changed. In our driving example above, the speed is the CV, the accelerator is the MV, and the external factors are the DVs. Models developed for this control process would tell you how far and how quickly to adjust the accelerator based on a change in any of the inputs.
It is important to note that MPC does not “control” CVs, per se, but rather, it determines feasible combinations of all MVs such that all CV steady state targets are at or within their operating limits. If more than one feasible solution exists, the economic information programmed into the controller will be used to choose the best feasible solution. This feature not only provides stability but pushes the plant to its economic optimum. If no feasible solution exists, the limits of the least important CVs are relaxed. Once the steady state targets of the MVs are calculated, MPC creates dynamic move plans for all MVs. The move plans are updated each execution cycle of the controller to account for changes in DVs.
At ACE, we know that getting started with advanced process control can be daunting. But we are here to help. We can collaborate with your process engineers to use their in-depth process knowledge, along with our deep knowledge of advanced controls, to design, test, build, and implement your multi-variable process control models.
Contact us today to discuss your control system needs in more detail.